How advances in computer vision provide clearer quality control
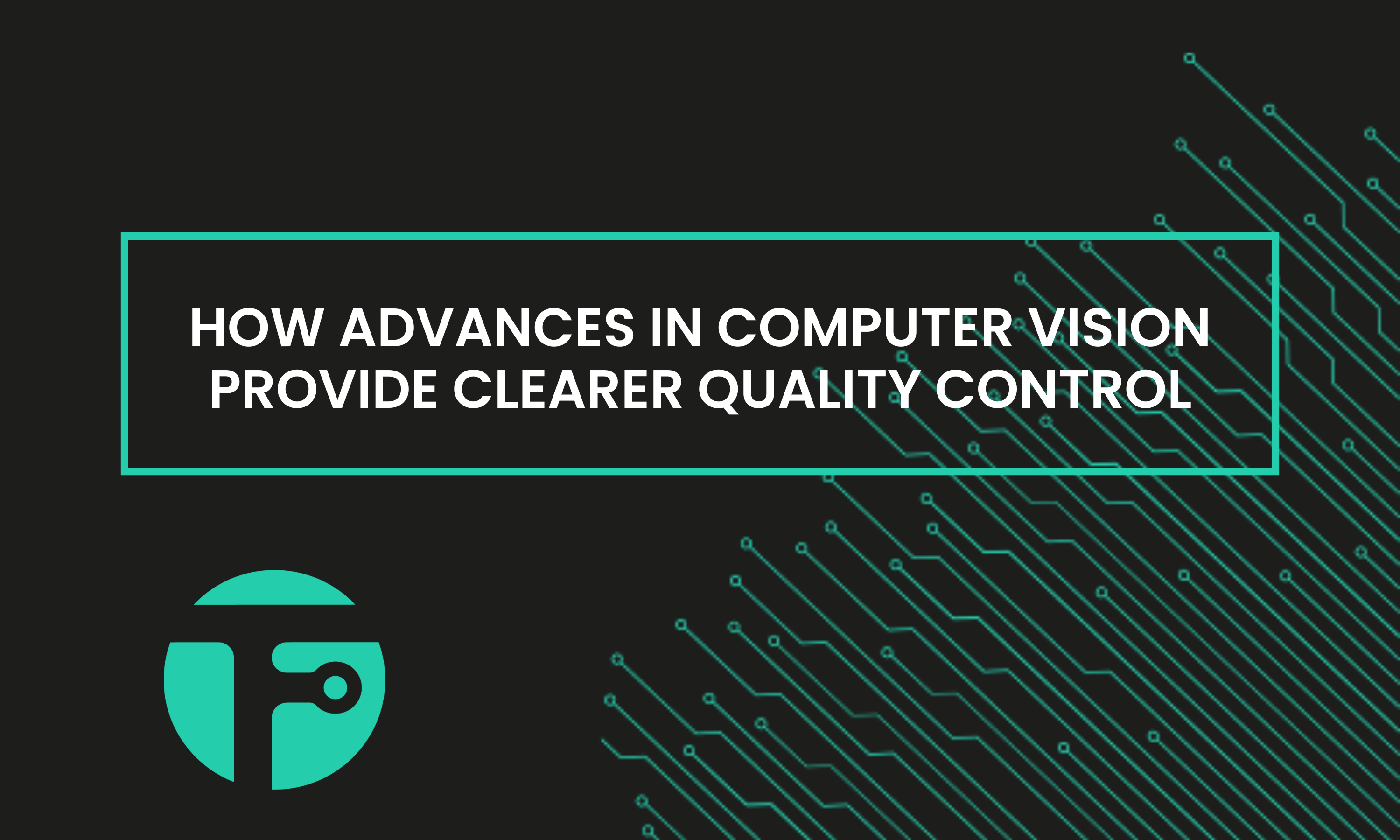
Deep learning, the process where a computer engages numerous tiers of “neural networks” to replicate how the human brain solves problems, came onto the scene only a few years ago. The technology is proving to be a significant step forward in quality assurance and process improvement for the companies who have already used it.
Prior to the advent of deep learning, automation controllers could only function within the strict boundaries of extremely constrained, rules-based logic. Full-fledged automation relied on the programmer’s ability to anticipate obstacles and predict outcomes. There was limited room to respond to changing conditions or adapt to demands.
Deep learning now enables a computer to proactively expand its knowledge base by extrapolating new information from relevant inputs and construct its own algorithms to do so. The most recent computer systems communicate with other information sources and draw conclusions for problem solutions from outside of themselves.
A closer look
In general, computer vision employs imaging technologies to collect pictures. It then uses computer-based criteria to evaluate, identify, analyze, and draw conclusions from the photos.
For example, AIDEAS is committed to making sure computer vision and AI become a reality for manufacturers all across Europe. Within the AIDEAS Project framework, researchers are conducting research on the role of 3D computer vision and AI in product inspection. The focus is on improving automatic defect analysis, integrating it with the AI-QA solution under AIDEAS to optimize the performance of various industrial equipment.
Computer vision combined with deep learning technology is already becoming a key component of many manufacturing AI systems. The high degree of interest comes from its potential to include cost-saving measures that are difficult to recreate, even with a larger staff.
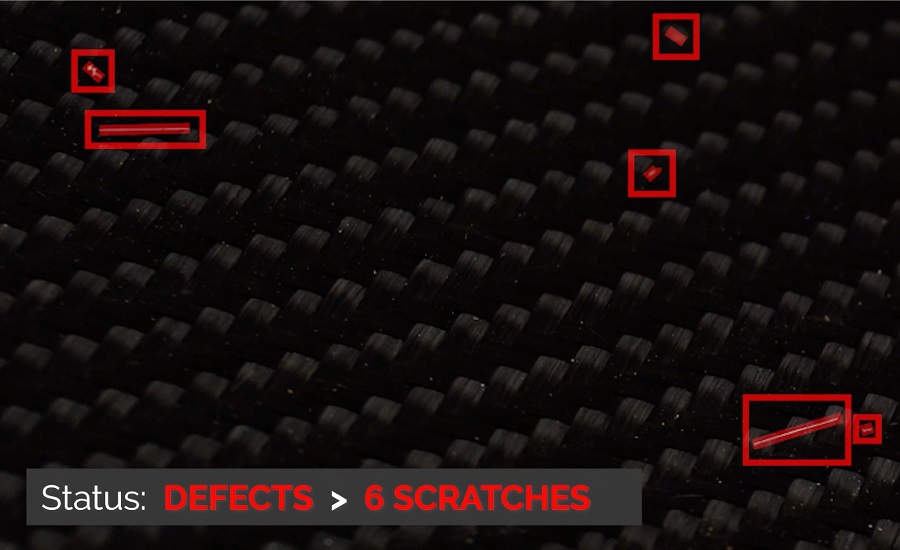
Image Credits: prakasitlalao – stock.adobe.com
For example, robotic welding systems have traditionally needed a human inspector to ensure the quality and integrity of welds as workpieces moved along the production line. However, this technique exposes producers to the danger that an issue would spread before it is recognized and addressed and increases the potential commercial repercussions.
The ability to perform the visual inspection earlier in the manufacturing process is crucial because it allows the engineers in charge of the robotic systems to make adjustments almost instantly, which reduces the impact.
Long-term analytics are also available to support efforts for continual development thanks to the priceless digital record that has been built over time.
Deep-learning sense
The benefits of these AI advancements go well beyond welding applications. Computer vision and deep learning have the potential to improve operations, quality, and safety while also driving financial value.
Cutting edge computer vision systems provide efficiencies in production or surveillance, establish ongoing enhancements, and quicken the application of those enhancements. Their data enables production teams to devise “work-to-zero” safety plans, which facilitates the implementation of zero-defect projects and gathers actionable insights.
Even in operations that need a high level of precision and repeatability, deep-learning AI may be very helpful. The potential for mistake is great when manufacturing processes for classical machine vision systems, bioengineering, metal forming and assembly, aerospace and automotive components, 3D printing, and related sectors rely on manual production or even manual oversight.
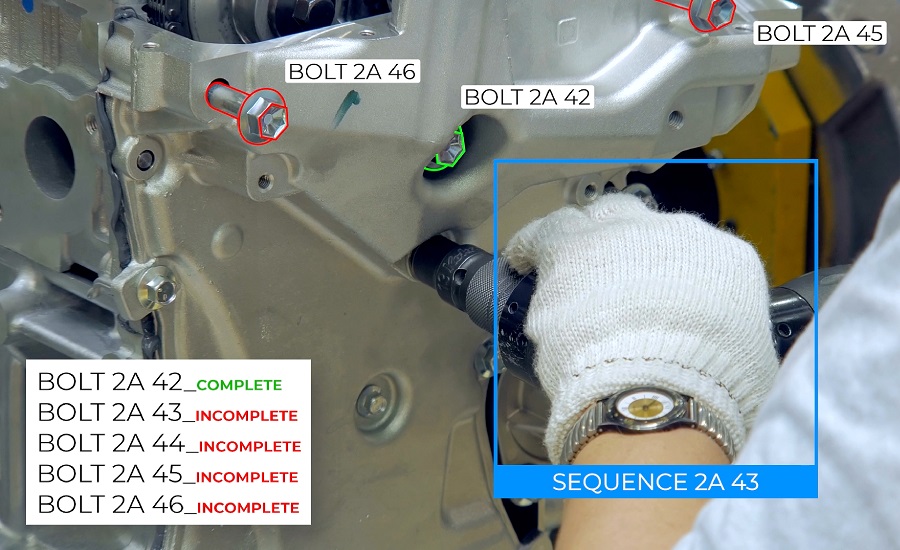
Imagery supplied by Andy_Star/iStock.com via Getty Images
The reliance on a human visual inspection component adds issues like as tiredness, repeated motion stress, a lack of 360° vision, and inherent subjectivity. Traditional machine vision systems must also account for their passive nature and limited ability to identify complex faults that may fluctuate in shape, size, color, texture, or location.
A system may fail to detect even a serious issue because of these technologies’ limitations. Blind spots can also be caused by original programming flaws or operator mistakes. Rules-based algorithms are incapable of dealing with variations like as lighting, color, or product placement, which exacerbates the problem.
Want to keep discussing more technologies like this? Join the smart manufacturing community and connect with over 2500 researchers, manufacturers and organizations from all over Europe.